Esther Chan's MSc Defence
Date and Time
Location
Teams: https://teams.microsoft.com/l/meetup-join/19%3ameeting_NTdmMzVjOWUtOGZmOS00Y2I1LWJlOWEtMzBiYjY1YzRiMTYw%40thread.v2/0?context=%7b%22Tid%22%3a%22be62a12b-2cad-49a1-a5fa-85f4f3156a7d%22%2c%22Oid%22%3a%22fbd28915-dda5-478f-8ecb-a3682dcf0c3a%22%7d
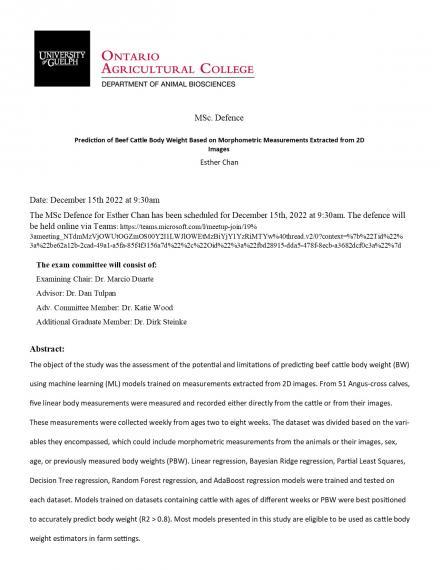
Details
The object of the study was the assessment of the potential and limitations of predicting beef cattle body weight (BW) using machine learning (ML) models trained on measurements extracted from 2D images. From 51 Angus-cross calves, five linear body measurements were measured and recorded either directly from the cattle or from their images. These measurements were collected weekly from ages two to eight weeks. The dataset was divided based on the variables they encompassed, which could include morphometric measurements from the animals or their images, sex, age, or previously measured body weights (PBW). Linear regression, Bayesian Ridge regression, Partial Least Squares, Decision Tree regression, Random Forest regression, and AdaBoost regression models were trained and tested on each dataset. Models trained on datasets containing cattle with ages of different weeks or PBW were best positioned to accurately predict body weight (R2 > 0.8). Most models presented in this study are eligible to be used as cattle body weight estimators in farm settings.